The personalization of medicine by machine learning for image interpretation
In this AI-hub project researchers are investigating the use of AI for image interpretation to improve the patient journey from diagnosis to treatment and follow-up. This will not only save time and money, but most importantly it will benefit patients with an approach that is more tailored to their individual circumstances, explains researcher Alessandro Sbrizzi.
Medical imaging is a foundation of modern clinical practice, enabling clinicians to gather valuable insights into the inner workings of the human body. However, the complexity of imaging modalities such as PET-, CT- or MRI-scans, or histology samples, presents a challenge in efficient and accurate analysis. ‘interpretation of these images is still done through visual inspection by highly trained medical personnel’, says Alessandro Sbrizzi, associate professor at the Computational Imaging group of the UMC. ‘This means it requires a lot of man-hours, and patients will sometimes have to wait longer before they can be helped or return to the clinic more often.’
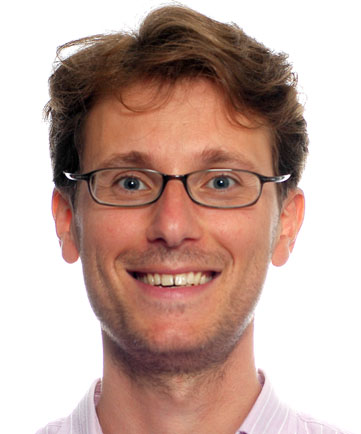
‘If we can better predict which patient would benefit from treatment, this means we could prevent unnecessarily subjecting a patient to a possibly harmful treatment, when this is not helpful’
– Alessandro Sbrizzi, associate professor at the Computational Imaging group of the UMC.
Artificial intelligence, especially deep learning, has significantly improved medical image processing and analysis in various tasks such as disease detection, classification, and anatomical structure segmentation. Sbrizzi and other Researchers at the UMCU and TU/E are investigating how AI can further be integrated into the medical imaging process, in order to improve all steps in the patients journey from diagnostics to treatment and follow-up.
Predicting patient outcome
Diagnostics is one area where AI could be useful, as accurate diagnostics improves personalized treatment planning. For example for patients with breast cancer. Tumor grade is an important predictor of patient survival. To determine this grade, you have to evaluate the histopathology images. However, there is significant inter-observer variation in breast cancer grading, and current methods require labour-intensive annotations.’ In a study from TU/e IMAG/e and UMCU Department of Pathology, a deep learning-based breast cancer grading model was developed and evaluated on whole-slide histopathology images. The model accurately distinguished low/intermediate versus high tumor grade and found a trend in the survival of the two predicted groups.
Determining when treatment is beneficial
Treatment is another area where AI can be helpful. In a project from the TU/e IMAG/e and UMCU Image Sciences Institute, Department of Oncology, researchers are looking at the possibility to use machine learning and image analysis, to predict which patients with advanced cutaneous melanoma are more likely to benefit from immunotherapy. This study investigates the added value of radiomics, a technique to quantitatively capture tumor characteristics on CT-imaging, for predicting clinical benefit. ‘If we can better predict which patient would benefit from treatment, this means we could prevent unnecessarily subjecting a patient to a possibly harmful treatment, when this is not helpful’, Sbrizzi explains.
Predicting post-operative risks
AI could also be used in patient follow-up, for example to reduce the amount of post-operative visits. A research group from the TU/e IMAG/e, UMCU Department of Ophthalmology and the UU Methodology and Statistics is looking at the possible uses of AI to predict which cornea transplant surgery patients need more frequent follow-up. Corneal transplant surgery is a common procedure in developed countries. One of the possible complications is graft detachment in the early post-operative weeks. To address this issue, an AI-driven solution proposes using high-resolution imaging techniques collected prior to and 1-day post-operation. AI is then used to identify patterns in local variations in corneal thickness that can predict graft detachment. ‘This prognostic tool will enable individualized tailoring of care, reducing the amount of post-operative visits for low-risk cases, while increasing vigilance for high-risk cases, leading to improved quality of care, reduced burden for patients, and healthcare cost associated with corneal transplant surgery.’
AI-hub
Working on this topic within the AI-hub had its advantages according to Sbrizzi. ‘We can share our different expertise and perspectives. And we can identify concrete clinical cases and evaluate the AI models in a clinical setting.’The different alliance partners also collaborate in education in this field. The UMCU and Tu/e for example, have developed a Master Program Medical Imaging. And the ewuu alliance also organnizes an annual workshop Deep Learning in Radiotherapy.
In March 2024, the AI-Hub is organizing a Hackathon AI in Personalised Medicine.
About the project:
Project title:
The personalization of medicine by machine learning for image interpretation
Research team:
Alessandro Sbrizzi (UMCU), Nico van den Berg (UMCU), Mitko Veta (TUe), Josien Pluim (TU/e)