When eating with friends or family , people tend to eat more than when they are eating alone. Scientists in this project use AI to investigate what social cues underpin this social facilitation of eating. ‘This could help for example with developing strategies to combat obesity’, says researcher Liam Hand.
The social context in which food is consumed affects what and how much is eaten ‘Individuals tend to consume greater amounts of calories when eating in groups with people they know, compared to when eating alone’, says Liam Hand, PhD student at Wageningen University and Research. This phenomenon is known as the social facilitation of eating. ‘The greater caloric consumption has also been shown to be sustained across multiple meals’, says Hand. ‘Which means that, even though an individual consumes a larger amount of calories in one meal, they do not counterbalance this by eating a smaller amount of calories at subsequent meals.’
‘Even though the social facilitation of eating is a well-observed phenomena, there have been very few studies which actually investigate why this happens’
It has been estimated that this behavior could lead up to four kilogram of weight gain per year, if a person was to eat one social meal per day. This will then have a great impact on the development of obesity, which is already a major health problem in society.
Hand: ‘Even though the social facilitation of eating is a well-observed phenomena, there have been very few studies which actually investigate why this happens. Social scientists have theories, but the actual social and physical cues which lead people to overeat in these social situations have never really been investigated. And this is where our project comes in.’
Step 1: capturing food real-time
The project is split into two distinct phases. Hand: ‘In the first phase we will develop an AI technology bundle that is capable of capturing various types of data to aid in our understanding of the social facilitation of eating. This includes developing a machine learning computer vision algorithm and software that is capable of capturing in real-time what foods are present in a meal. Convolutional Neural Networks (CNNs) are widely considered the best machine learning algorithm/architecture for classifying foods from images, which can then be extended to real-time video. In order to train a CNN though, a great amount of data is needed. Fortunately, we can leverage already existing datasets and CNN models to create our own unique model. We have already identified the most common foods that Dutch people eat via the results of the Dutch National Food Consumption Survey 2019-2021. We have since created our own dataset on a subset of these foods, using already existing images from current food datasets, and web scraping of social media.’
‘We hope to determine what social and physical cues are responsible for the social facilitation of eating phenomena‘
‘We are currently using transfer learning (essentially taking what a previous neural network has learned, and applying it to a different model/domain), from existing CNN models, and evaluating which is the most accurate for use with our own data. Since recognizing food is the first step for the AI. The AI will be able to do this on its own, with zero human intervention’, says Hand. ‘To achieve this we will integrate the computer vision software with a “Smart Tray” that has already been developed at Wageningen University and Research (WUR). This tray has sensors capable of recording the exact weights of foods taken from anywhere on the tray.’
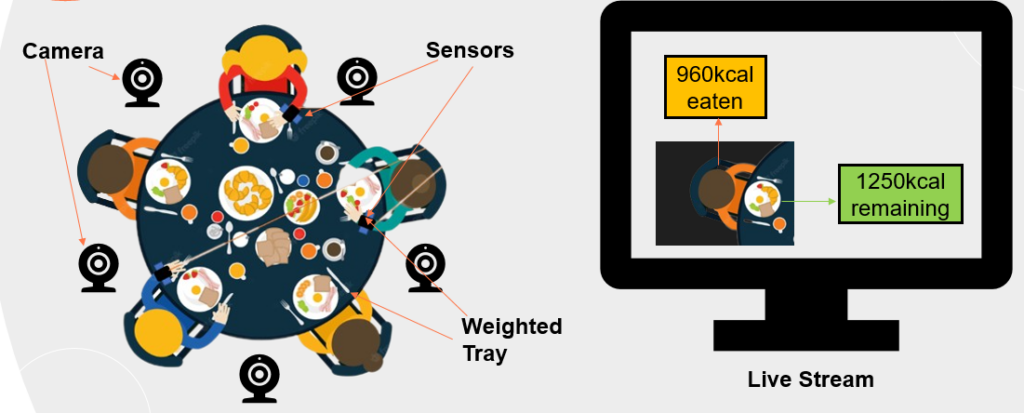
When combined with the computer vision software, it will give us the ability to record in real-time for example what foods are present on the tray, how many calories have been consumed by individuals and how many calories remain on the tray. Next, we will work on further integration of other sensors capable of recording various sociometric data into this technology bundle. We have identified sensors for example which will help us record data such as face-to-face interactions within a group, speech activity, proximity of individuals to friends in the group, etcetera. These will be used in conjunction with the technology, therefore providing us with a holistic collection of data sources to aid us in our investigation of the social and physical cues which contribute to the social facilitation of eating.’
Step 2: live trials
In the second phase of the project the team wants to use the AI technology bundle in live trials. ‘In this stage, we will have developed and validated our technology, and will be able to run a number of live trials for the collection of all the aforementioned data. We aim to have a number of trials where individuals eat with friends, and then on a separate date eat alone. We will collect all the data, and then the data analysis stage will begin.’
‘We are hoping to be one of the first groups which will have looked at all these data sources together, and with the help of AI, be one of the first groups to determine what social and physical cues are responsible for the social facilitation of eating phenomena. This could for example help to develop future interventions to prevent individuals from overeating in social eating scenarios and thereby help combat obesity. It is important to note that social eating itself shouldn’t be discouraged, because of the positive benefits it has on mental health, therefore other strategies to combat the potential weight gain and development of obesity through the social facilitation of eating should be developed. I also believe that the insights of this project might help people that suffer from undereating and malnourishment by developing strategies to encourage eating.’
ABOUT THE PROJECT:
Project title:
Deep learning on multimodal inputs (video, sensors) with use case: individual feeding behaviour from consumption data
Research team
Ricardo Torres (WUR), Guido Camps (WUR), Liam Hand (WUR), Albert Salah (UU)